How to Use Data to Predict Trends in Marketing
Learn what simple linear regression is and how it helps predict trends in marketing by identifying relationships between variables for better decision-making.
Predicting future trends in marketing is essential for making informed decisions. You can uncover actionable insights such as estimating sales growth, understanding customer behaviour, or optimising ad spend by analysing marketing data. One simple and effective method for this is simple linear regression, a statistical technique that identifies relationships between variables to make predictions.
If you’d like to learn more about variables, view the variables in marketing guide.
Let’s explore how simple linear regression works, why it’s useful for marketers, and how you can start applying it to predict trends in your marketing strategy.
What is Simple Linear Regression?
We use simple linear regression as a statistical method to identify relationships between variables. It helps answer questions like, “How does increasing my ad budget impact sales?” or “What customer age group is most likely to purchase our product?”
We use it to predict a dependent variable (outcome) based on one or more independent variables (factors influencing the outcome).
Unlike more complex regression models that use multiple variables or non-linear relationships, this method, known as simple linear regression, uses just one independent variable to predict the dependent variable.
Through the analysis of historical data, linear regression creates a straight line that intersects the data points on a graph. This line, called the “line of best fit,” represents the relationship between the variables. If your data shows that increasing ad spend leads to higher sales, linear regression provides a formula that can estimate sales for any given budget.
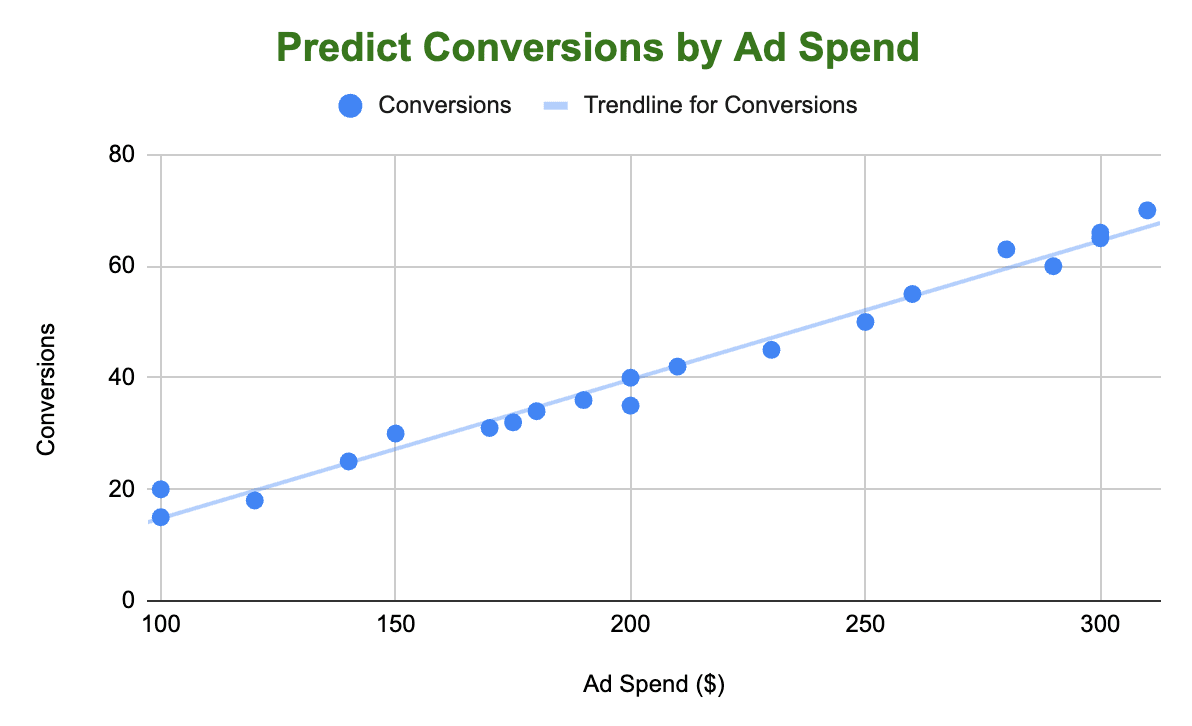
Why is Linear Regression Useful in Marketing?
Predictive analytics in marketing often starts with methods like linear regression to uncover trends. Marketing decisions often involve answering questions such as these.
- Which campaigns are driving the most revenue?
- How can I allocate my budget to maximise returns?
- What customer behaviours predict future purchases?
Linear regression can uncover actionable insights in your data, helping you make data-driven decisions. Here are some examples of its application in marketing:
- Predict Sales: Analyse how ad spend *correlates with sales figures.
- Optimise Campaigns: Determine which channels deliver the best ROI.
- Anticipate Customer Needs: Forecast which products might be popular in the next quarter.
*Correlation measures the strength and direction of a relationship between two variables. A positive correlation means that as one variable increases, the other tends to increase. A negative correlation means that as one variable increases, the other tends to decrease. If there’s no correlation, it means the variables don’t show any consistent relationship.
By using linear regression, you can make smarter decisions and maximise the impact of your marketing efforts.
How Simple Linear Regression Works
Linear regression starts with two types of variables:
- Dependent Variable: What you want to predict (e.g., sales).
- Independent Variable(s): The factor(s) that influence the outcome (e.g., ad spend, email campaigns).
When applying linear regression, the first step is to plot your data points on a graph, creating a scatter plot. This plot visually represents how your variables interact. Each dot on the scatter plot corresponds to a data point. For example, if you’re studying how ad spend relates to sales, one dot might represent the sales revenue for a specific amount spent on ads.
Linear regression then calculates the line of best fit, which is the straight line that best represents the relationship between your independent and dependent variables. This line is what allows you to make predictions based on your data.
For instance, if the scatter plot shows that higher ad spend generally leads to higher sales, the line of best fit will slope upward. Once this line is calculated, you can use its formula to estimate sales for any given ad budget.
Beginner-Friendly Examples of Linear Regression
Let’s examine two real-world applications of linear regression to make it easier to understand.
1. Predicting Sales from Ad Spend
You own an online store and want to understand how advertising impacts sales. You collect data on your monthly ad spend and the corresponding sales for each month.
Dependent Variable: Sales (the outcome you’re trying to predict)
Independent Variable: Ad Spend (the factor that might influence sales)
With simple linear regression, you can find the relationship between ad spend and sales. For example, you might discover that every additional $100 spent on ads leads to a $500 increase in sales. This relationship allows you to predict sales based on future ad spend budgets.
2. Forecasting Email Open Rates
You’re running email marketing campaigns and want to optimise the subject line length to improve open rates. You gather data on the subject line length and the open rates for each email campaign you’ve sent out.
Dependent Variable: Email Open Rate (the outcome you’re trying to predict)
Independent Variable: Subject Line Length (the factor influencing the open rate)
You could discover that emails with subject lines between 5 and 7 words tend to have the highest open rates after creating a linear regression model. This helps you forecast the expected open rate based on the subject line length of future campaigns.
Limitations
While linear regression is a powerful tool, it’s not perfect. Here are some common limitations:
- Assumes Simplicity: A key assumption of linear regression is that the relationship between the variables is linear, meaning it can be represented by a straight line. This means that as one variable changes, the other changes at a consistent rate. This might not always exist.
- Data Quality Matters: It requires clean, relevant data for accurate predictions.
- Ignores External Factors: Factors outside the dataset, like market disruptions or changing customer preferences, are not accounted for.
- Influence of Outliers: Extreme values can skew results, making predictions less reliable.
These challenges mean that while simple linear regression is a great starting point, it’s not a one-size-fits-all solution. However, it offers a straightforward way to forecast trends in marketing and refine your strategies. You can make better decisions about budget allocation, customer targeting, and trend forecasting by understanding the relationships between variables.
Ready to take the next step? In the next guide, I’ll explain how to validate your model to ensure accuracy and reliability.